Evgeni Gousev
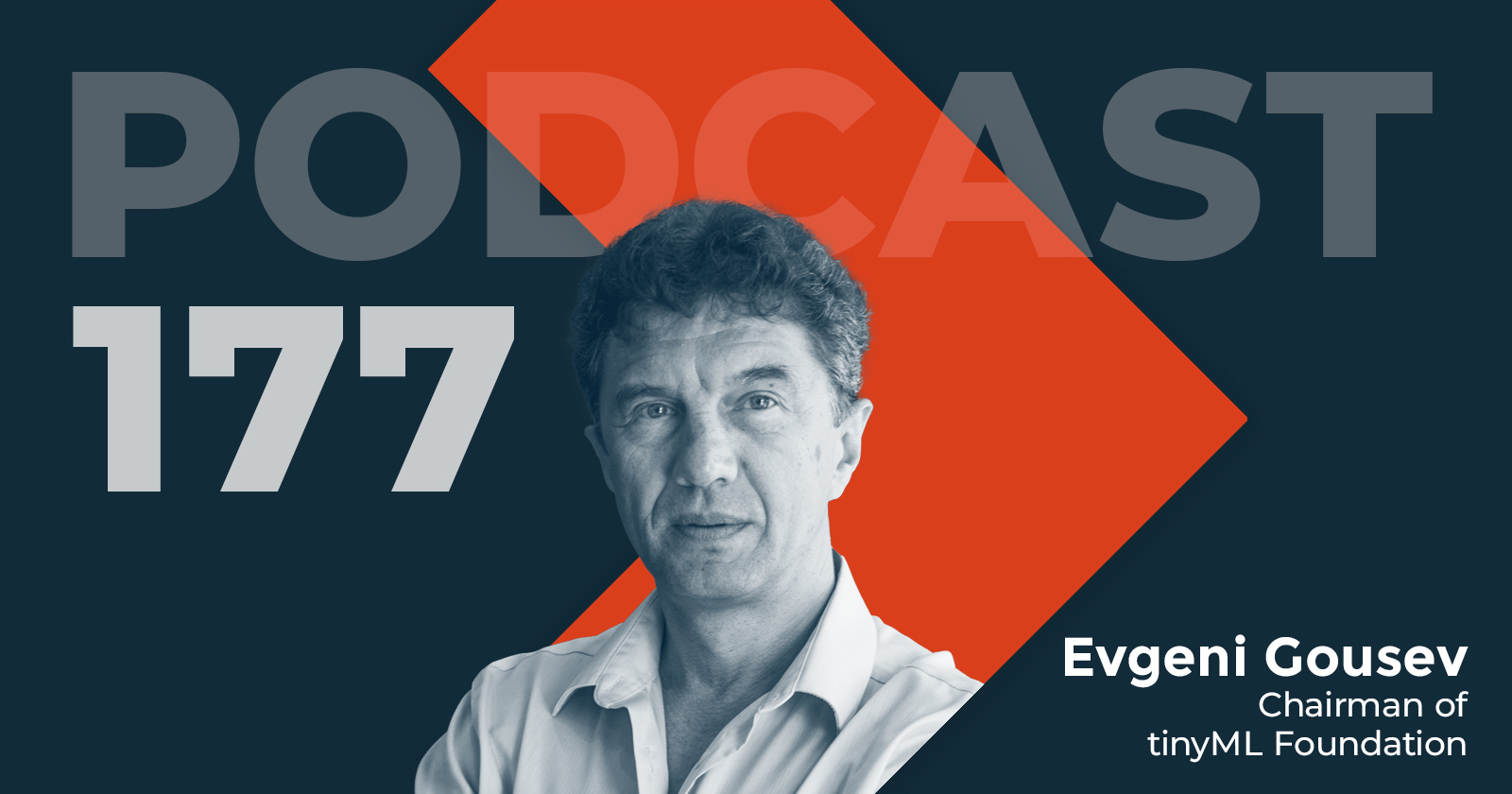
TRANSCRIPT
Ken: Good day, and welcome to Episode #177 of Momenta's Digital Thread podcast. I'm pleased to welcome Evgeni Gousev, Chairman of the tinyML Foundation, a nonprofit organization with the mission to accelerate the growth of a prosperous and integrated global community of hardware, software, and system scientist, engineers, designers, products, and business application people are developing leading-edge energy-efficient machine learning to compute.
Evgeni is a senior director of engineering at Qualcomm Research in Silicon Valley, where he leads the R&D organization. He's in charge of the company's ultra-low-power embedded computing platform, which includes always-on machine vision AI.
He joined Qualcomm technology in 2005 after working at IBM's TJ Watson research center, where he oversaw advanced silicon technology projects. Evgeni worked as an academic professor at Rutgers University and Hiroshima University from 1993 to 1998. He has a master's degree in applied physics and a Ph.D. in solid-state physics, and he has co-edited 24 books and published 163 papers, as well as being an inventor on more than 60 issued and pending patents. Evgeni, thank you for joining us on the Digital Thread podcast.
[00:02:05]
Evgeni: Thank you for the introduction, Ken; it's a pleasure to be here. Also, thank you for assigning me episode 177; 17 and 7 are two of my favorite numbers.
[00:02:20]
Ken: I am a numerology guy myself, and of course, we just passed 2,22,22 a couple of weeks ago, so it made for an exciting day, but I really appreciate you taking the time to join us, and that mouthful of a bio says so much about who you are and the experience base you have. I am happy to have you join us today and especially talk about a topic that is near and dear to our heart in the form of tinyML.
Let's start with my favorite question - What would you consider your digital thread? In other words, what are the one or more thematic threads that define your digital industry journey?
[00:02:54]
Evgeni: Throughout my professional life, I've worked on a variety of projects, industries, and technologies, starting with “hardcore” silicon, and progressing to areas such as MEMS, displays, and user interface technologies, among others, but these days I'm very fortunate and extremely enthusiastic about tinyML technologies.
TinyML technologies are a set of hardware, software, and algorithmic techniques that enable and allow you to do machine learning in artificial intelligence at extremely low power, very low power, and that enable battery-operated devices in terms of performance.
The cool thing about tinyML is that it's super interesting from a technological standpoint, but I'm also very interested in the impact that technology can have on our lives as consumers and citizens. I believe they are extremely powerful, and we are at the start of a major tinyML revolution that will transform our lives.
[00:03:58]
Ken: I couldn't agree more. In fact, Pitchbook published an interesting perspective on tinyML last year and had very good things to say, very much along the lines with what you have as well, looking at it as a major theme for potential investors in this space as well.
You are certainly uniquely qualified to talk about this given that you have been in the leading edge of R&D for almost 25 years now, as you mentioned, silicon design, MEMS, the human-machine interface actually and now ultra-low power edge including machine vision.
If you had to boil all of this down into three key insights about those technologies and your time, what would those be?
[00:04:40]
Evgeni: I think 25 years is a long time, but it feels like yesterday, and in those 25 years, I've had the privilege of working with some of the brightest people on the planet on extremely difficult technologies.
Working with extremely talented people on mission-impossible technologies leaves you with scars, and I've accumulated quite a few over the years. I've had my ups and downs, as have many of us, and I've distilled these lessons into three main points.
The first one I learned was at IBM about 20 years ago. Back then, we were working on advanced Silicon technologies. When I joined IBM, we were developing 180-nanometers technologies, and when I left IBM 7 years later, we were working on 45- nanometers technologies and no one believed there would be technologies beyond 45nm because of optical lithography limitations. So what do you do if you are at the physical limits of things?
What I took away from this lesson was that, while we are now at 5- nanometers production, as what we did at Qualcomm, a factor of ten less was unthinkable 15 years ago. My first takeaway from my IBM days was that technological progress is virtually unstoppable. If there is a driving force there is a group of great talent in the world, and we do have a lot of talent in the world, all companies, all countries, and all disciplines combined, then technological progress will never stop.
Even if you feel like you've reached a dead end or a brick wall, there are always solutions, and that's something I keep reminding myself of whenever we run into a problem or a roadblock. I recall my IBM days, there was always a solution.
It is not immediately apparent, but after some time, it becomes apparent, and we solve the problem; no matter what the problem is, there is always a technical solution or a way around it. So that is my first lesson.
Second, since my early days in the industry, I've had the good fortune to work on some very difficult problems, as I mentioned earlier. As a result, to find a solution, we had to develop unique disruptive technologies. What I learned from those days is that disruptive technology is a lot of fun to work with. It's not easy, but it's a lot of fun to go through all the “bumps” and come up with a solution. What I learned, and what I believe is a valuable lesson for startups entering this field, is that to be successful with disruptive technologies, you must aim for a 10x improvement in at least one of the parameters: you must develop something 10x better in terms of power, performance, or user experience.
This 10x factor is very important to me when I start a new project, whether it's at Qualcomm or working with people outside of Qualcomm; this 10x factor is my guide because it takes time to develop technologies, and by the time you're working on something new everything else also has advanced. I believe you are fortunate if you get 2X to 3X. So, my second lesson is that your starting point should have a 10x factor at the very least.
Lesson number three is that to be successful with the technologies that we are developing, particularly in the AI and tinyML spaces, you must look at your systems and solutions holistically because this gives you the most competitive advantage and edge.
You must be familiar with cutting-edge hardware technologies, particularly tinyML hardware accelerators and silicon tools, and you must be able to understand and design the most efficient algorithms to run on this hardware. Furthermore, if you don't have good software that can deliver this benefit to the customer, it's a waste of time.
You need to look at the systems and products you're developing holistically, from all angles. I've been promoting this to younger generations recently. My physics background, which includes a degree in physics, solid-state physics, and microelectronics, has been extremely beneficial to me. Because real physicists are trained to look at systems by connecting laws, by connecting dots and laws of physics, my physics background gives me this holistic way of looking at things.
These are the three most important lessons. There are numerous others. I'll summarize the first one: there are always solutions to all problems; just don't give up and choose to work with smart people to find them. The 10x factor should be your starting point for new disruptive technology, according to lesson number two. Lesson three is to look at the solutions from a holistic standpoint; in the tinyML space, this means looking at the hardware, the algorithm, the software, the use cases, and everything. That is my only response to this fascinating question; thank you for posing it, Ken.
[00:10:10]
Ken: Great, so be tenacious and aim high at least 10x and engineer holistically. Three great learnings here, and I am sure Gordon Moore would agree with you on 10x improvements there in Moore's law. Let's dig into tinyML; you started to talk about it a few minutes ago, but what is it, tell us a little bit about the origin story and really what attracted you to this space?
[00:10:36]
Evgeni: My tinyML journey began in 2014, and it began with a 10x, or, at the time, a 100x - thing. We were really challenged at Qualcomm back then to do new disruptive things, and one of the most difficult problems we were trying to solve was computer vision. Cloud-based CV was state of the art then if you wanted to get recognition of a person, a car, or anything else. Take an image sensor, particularly these high-resolution image sensors, which can see with tons of power consumption, especially security cameras, as an example.
At that time, you take a picture of a person or a car, which requires a lot of energy or power, typically in the hundreds of milliwatts, and you need the transmitters to get to the next level where you do processing, such as the CPU, GPU, or DSP type of core, and then you do process and the algorithm there.
They weren't very efficient back then because they were designed for big CPUs and the cloud, which used a few hundred milliwatts. When the transmission part is added up, you get half a watt of power. We put ourselves to the test by asking, "Why is it so high?" We wanted this computer vision to be always on, and our definition of all always-on technologies at Qualcomm was less than 1 milliwatt, but we're talking about more than a factor of 100, so 1 milliwatt versus 500 milliwatts because I believe 1 milliwatt was the magic number in the cellphone market. For cell phone technologies to qualify for always-on, such as accelerometers and touch sensors from the screen, they had to be less than 1 mW.
We asked ourselves, "Why is it impossible to have an always-on vision?" When we first started this project, it was like, wow, there's no way we'll be able to do this - to the point that I made earlier, point number one when you hit a major roadblock - my team and I had been thinking about this problem for 6 months - after 6 months, we finally saw the light at the end of the tunnel. The key for us was to take a holistic approach to the problem, i.e., why is the sensor consuming so much power, why is the algorithm consuming so much power - in either case, we can design a better processor, a better computer to run these algorithms.
We came up with this one-of-a-kind solution after a year, and it was like a magic - one type of number opened a whole new area for us to innovate and commercialize this technology. We basically enabled always-on vision for the first time in the industry – this was a project we started in 2014.
That led us to the tinyML space because we developed very efficient hardware that could run efficient algorithms that could do machine learning back then, and that is the definition of tinyML.
The more we thought about it, the more we realized how disruptive it would be; for the first time, you can enable so many interesting use cases and run them on battery-operated devices, which is hugely disruptive. That is essentially how the tinyML movement began, with us talking to its partners and eventually leading us to where we are now.
[00:14:20]
Ken: You have cofounded tinyML foundation in 2018; tell us a bit about the goals of this foundation?
[00:14:26]
Evgeni: That, I believe, is ingrained in my DNA as a collaborative person. I am a strong believer that today, to develop technologies and develop them fast you really need to partner with the right people. You must create an entire ecosystem. That is precisely how the tinyML foundation began in 2018. We started talking to our customers about it, and one of them was Google, specifically Pete Warden, who was one of the key people there. I paid Peter a visit to his new, modern office one day. We got to talking about what we were going to do about it. At Google, Peter had begun some TinyML-related activity. We started working on this idea for a couple of years and quickly realized it was going to be big.
As a result, we made the decision to create something and propel this ecosystem forward. We took baby steps at first, organizing a workshop and inviting people. Then one day, we met with Stanford's Boris Murmann, as well as representatives from Arm and other companies. The meetings began with a small group of about 5 people. We considered it would be a success if we could get about 30 people to show up. We began publicizing the event, and within three months, we had over 200 people at our door. It was a great indicator and testimony for us that the community and ecosystem are ready for something like this. That was the catalyst for the creation of a global tinyML ecosystem. We formally organized it as a nonprofit organization from scratch, starting with a couple of hundred people.
We had about 200 people show up at our door at the 2019 Summit, and now we're talking about ten thousand. The tinyML community is truly international. There are approximately 44 groups in 34 countries, spanning the globe. The tinyML community is made up of a lot of energy and talent. It's a way to democratize AI gateway to provide technology to people who want to use it to solve problems in a cost-effective and quick-to-market manner, and that's what keeps us going.
It's about how we get this technology into the ecosystem, to end-users, and how we educate people about it. How do we enable all these exciting new applications that will game-changers not only in the commercial space but also in the social and information goods space? So, it's been three years, and it's been an incredible journey for this community. In three weeks, the tinyML summit, our annual flagship event, will take place in the Bay Area. Because this will be an in-person event, I am very excited. We have 44 company sponsors signed up as of today, and we will have over 30 strategic partners. This is a fantastic ecosystem, and the academic community is very supportive.
[00:17:50]
Ken: Well, I couldn't agree more with you about the impact and the potential of tinyML. In full transparency, we are of course big fans at Momenta having invested in 3 rounds for Edge Impulse, Zach Shelby company which is the leading tinyML platform. Zach and Edge Impulse are cornerstones of the foundation as well.
You mentioned the sponsors and associated partners; what’s what are some of the other more notable companies that are part of the foundation at this point?
[00:18:19]
Evgeni: Since its inception, Edge Impulse has been active. In fact, before we started the foundation, I had lunch with Zach Shelby, who was still at Arm. He was also thinking about how big tinyML was going to be. He later founded Edge Impulse, while Pete and I founded the TinyML Foundation. We work well together, and I believe we all appreciate organizations like Momenta, which recognized this early on and is assisting companies like Edge Impulse and others in growing their businesses in this space.
To answer your question, I would say there isn't one company that stands out above the rest. Because the tinyML ecosystem is so diverse, every single player is important. Every single player brings something unique to the table.
We have businesses that specialize in hardware, software, automation tools, and architecture. People who are working with analog computers, compute in memory, firmware updates over the air. People working on these technologies, as well as companies such as Sony and SAP, will be present, as will strategic partners such as Google, Qualcomm, NXP, ST, Renasas, and other major silicon firms.
But, once again, I believe that the diversity of tinyML's worldwide community ecosystem is more important than the big names. Every organization contributes their skills, expertise, and knowledge of technology to finding industry pain spots and then working to solve these issues using their talents and knowledge of technology.
We are very proud of the fact that we built it from the ground up; diversity and inclusion, as well as being open and transparent and truly industry-driven. Focusing on high-quality “stuff” is part of our DNA. So, to return to my original answer, I cannot single out one organization, as all of them are important. Momenta obviously is aware of Edge Impulse because you sponsor and fund them.
But I would say that every single player, big or small, is important and adds value, and this list of companies is rapidly expanding. Every week, more businesses join because they recognize the value, see the opportunity, and appreciate the culture and ecosystem that we promote.
[00:21:17]
Ken: I would have expected nothing less than you are giving me the egalitarian answer given that you are an ecosystem builder and, I appreciate the fact you are. I am going to put you on the limb one more time in that I am curious about what are some of your favorite use cases or applications of tinyML that you have seen out there?
[00:21:39]
Evgeni: If you go back to the basics of TinyML, tinyML is a set of technologies that can connect the digital and physical worlds. So, in the end, I believe that is the best way to design systems and data analytics at the intersection of the physical and digital worlds.
That is why it is so powerful and has so many applications and opportunities because, effectively, there are all kinds of sense modalities in the physical world, right? We have a lot of information in the physical world coming from the audio types of signals, right? I believe human perception, about 11 percent of human perception, is through our ears.
The other major modality is vision, which accounts for about 80% of human perception. As a result, many technologies rely on visual responses, and we also have other types of sensors, such as temperature sensors, vibration sensors, infrared sensors, and other types of sensors. The beauty of tinyML is that it collects all this data from the physical world and analyzes it locally at the very edge, right where the physical world meets the digital world.
When it comes to using cases, and particularly my favorite use cases, they can be divided into a variety of categories based on modality, and I believe some market analysts do as well: vision-based applications, audio types of applications, and other sensor types of applications, such as temperature level sensors.
There are many applications in all these spaces, but the ones that are gaining traction now are industrial IoT. Specifically, with many companies doing things like predictive maintenance and anomaly detection. For example, you can attach a tinyML device to monitor a factory, or engine of aircraft, or your car engine or a pipeline and train this system to detect the abnormal type of behavior of this machine or engine.
This is very powerful because it allows companies to save a lot of money on maintenance, repairs, and other similar expenses, and it also provides real-time analytics to allow them to do a lot of things. So, there are many applications in audio, such as keyword detection, and there are also unexpected cases where you can train your models to distinguish certain sounds, such as breaking glass in your house, which you can detect if someone breaks in. You can train your tinyML audio system to do it or to distinguish gunshots from passenger car sounds.
Then obviously in the vision space, there are thousands of applications and in all industries, such as retail, you can monitor and track items in the store, empty shelves, and customer attention to specific items, such as how much time they can spend in front of a specific item on the shelf. Is it something they like, or something they don't like?
As a result, you can perform a lot of real-time analytics, which is very useful for these businesses or in logistics and transportation or asset tracking. When you really need real-time data and want to do it on a battery like a coin cell battery while also keeping costs down. So, when you deploy a solution like tracking a packaging box, you want to make sure that the system isn't adding a lot of extra costs while also providing real-time analytics.
These are some examples and use cases of industrial IoT; there are many others around us, such as in smartphones; people are starting to go metaverse now, which is a controversial topic in my opinion; why do we need metaverse if we have such a beautiful universe? But that is a topic for a separate podcast.
What exactly is the metaverse? You really need a bunch of sensors all around us to assist, guide, and augment human senses, and that's exactly what tinyML provides. AR and VR headsets are a classic example of this because these devices require a lot of compute power but are constrained in terms of form factor, thermal management, battery size, and compute. This is where tinyML comes in and offers a unique solution.
It's almost as if we're talking about a 10x advantage. tinyML, I believe, can provide a 10x improvement in AR VR and be disruptive for many use cases. This is a whole metaverse area for enabling all smart devices and doing so in a very energy-efficient manner.
I'm also very passionate about using tinyML for Good; giving technology back to society and giving this technology back to people because there are so many ways that the technology can be used to improve our lives around us, such as wildfires in California, which, as you may know, are becoming increasingly common in the United States and around the world because of climate change.
If you put these devices in a forest, you can get real-time data and analytics on what happens there if there is smoke, fire, or any kind of increased temperature, or any kind of increased infrared activity.
So really get this real-time data and use it for good or in education. We have a tinyML company in Kenya, Africa. I love this example because it shows how tinyML technology can be used to solve global problems. This company is called Ibees, after bees, the creatures that produce honey, because, according to what I've learned, the honey industry in Kenya is quite large. There are about 90,000 farms there, and they obviously produce a lot of honey.
The problem for them is that they are losing 30% of their honey due to diseases in the beehive, in the bee family, or because people are stealing them, or because there is disruption. They're basically losing 30% of their revenue on their products because they don't have this kind of feedback.
So this company developed a tinyML device with temperature and audio type sensors inside the beehive, to monitor the beehive, the environment, and the conditions of the beehive in real-time, similar to the motor in an industrial IT environment, where you look for abnormalities and monitor abnormalities, but you can do it in this kind of beehive environment, and it helps them save a lot of money, and it brings technology to them.
This is a great example because it demonstrates a few things: how simple it is to use tinyML technologies, how low the barrier is for people in the developing world to use it, the cost of this technology is quite low, and then we have a community of people all over the world who are passionate about using this technology to solve problems in their communities, and that is what makes tinyML super powerful.
There are silicon technologies available, and companies in the tinyML ecosystem design boards, development kits, and other similar items, so if you have a problem that you want to solve, tinyML is the way to go for doing so at extremely low power.
We held a tinyML vision challenge contest last year. We challenged our global community to come up with new tinyML vision application cases. We received 500 entries and chose five winners from them. A team of 5 to 9-year-old kids from Toronto, Canada, was one of the honorary winners; they had created a tinyML application for a smart bird feeder.
Their goal was to tell the difference between a bird and a squirrel because squirrels seem to eat a lot of food from these bird feeders. This example demonstrates how simple tinyML solutions are to utilize. So even in this case, primary school kids were able to design their answers and build a model over the summer to demonstrate that you can distinguish a squirrel from a bird.
This is what keeps me excited about tinyML and hopeful about the technology. With such a large global community and so many use cases, I believe we are looking forward to seeing how this technology can be used both for good and to improve people's lives in general, as well as for commercial purposes.
To summarize this long story of application cases, the TinyML foundation's mission or vision statement is "We see a new future with trillions of intelligent devices enabled by tinyML that work together autonomously to create a better, more sustainable, and healthier environment for all of us." So that's a very powerful vision statement, and it's not science fiction; tinyML can achieve that, and we're seeing it happen right now.
[00:32:00]
Ken: Excellent, you are very passionate about it, which is great, so to learn more about tinyML and the tinyML summit coming up in three weeks, go to www.tinyML.org. Evgeni, thank you for sharing this time and insights with us today.
[00:32:20]
Evgeni: It was an honor and privilege for me to be here today, Ken, to share the latest and greatest from tinyML, as well as what's going on in the world of technology and applications. Go to tinyML.org if you're interested in joining us. There is a wealth of information there, or you can connect with me on LinkedIn, and we will be happy to help you with any questions or opportunities, and so on. Thank you for inviting us to speak with you today; it has been a pleasure.
[00:32:53]
Ken: As well, thank you so much. So, this has been Doctor Evgeni Gousev, Chairman of the tinyML foundation, dedicated to developing leading-edge energy-efficient learning computing and, as I might add 10x.
Thank you for listening, and please join us next week for the next episode of our Digital Thread podcast series. Thank you and have a great day. You've been listening to the Momenta Digital Thread podcast series. We hope you've enjoyed the discussion. And as always, we welcome your comments and suggestions. Please check our website at momenta. one for archived versions of podcasts, as well as resources to help with your digital industry journey. Thank you for listening.
[The End]
[The End]
Connect With Evgeni Gousev via LinkedIn
What inspires Evgeni:
Inspiration comes from three different sources. First and foremost from PEOPLE. I gain a great deal of knowledge and inspiration from the people with whom I interact on a daily basis. Regarding those who have passed on, I enjoy reading books and watching documentaries about strong characters and pioneers who were considered "outliers" at the time but then made a significant impact on history, despite all odds. Second, as a natural-born technologist, I enjoy tackling difficult problems and developing disruptive technologies. It keeps me energized. Thirdly, I am energized by mother nature. Nothing compares to the majesty of the ocean, a forest hike, or a mountain backpacking trip. Nature teaches us so much.
About tinyML Foundation:
Machine learning technologies and applications that use very little power, often in the mW range or less, are called tiny machine learning. The tinyML Foundation, with over 10,000 members, sets the technical direction and strategy for the ecosystem, facilitates collaborations and partnerships, promotes sustainability, and educates critical audiences.
Tiny machine learning is a rapidly growing field of machine learning technologies and applications that include hardware (dedicated integrated circuits), algorithms, and software capable of performing on-device sensor (vision, audio, IMU, biomedical, etc.) data analytics at extremely low power, typically in the mW range and below, enabling a wide range of always-on use-cases and targeting battery-operated devices.