Gary Chandler
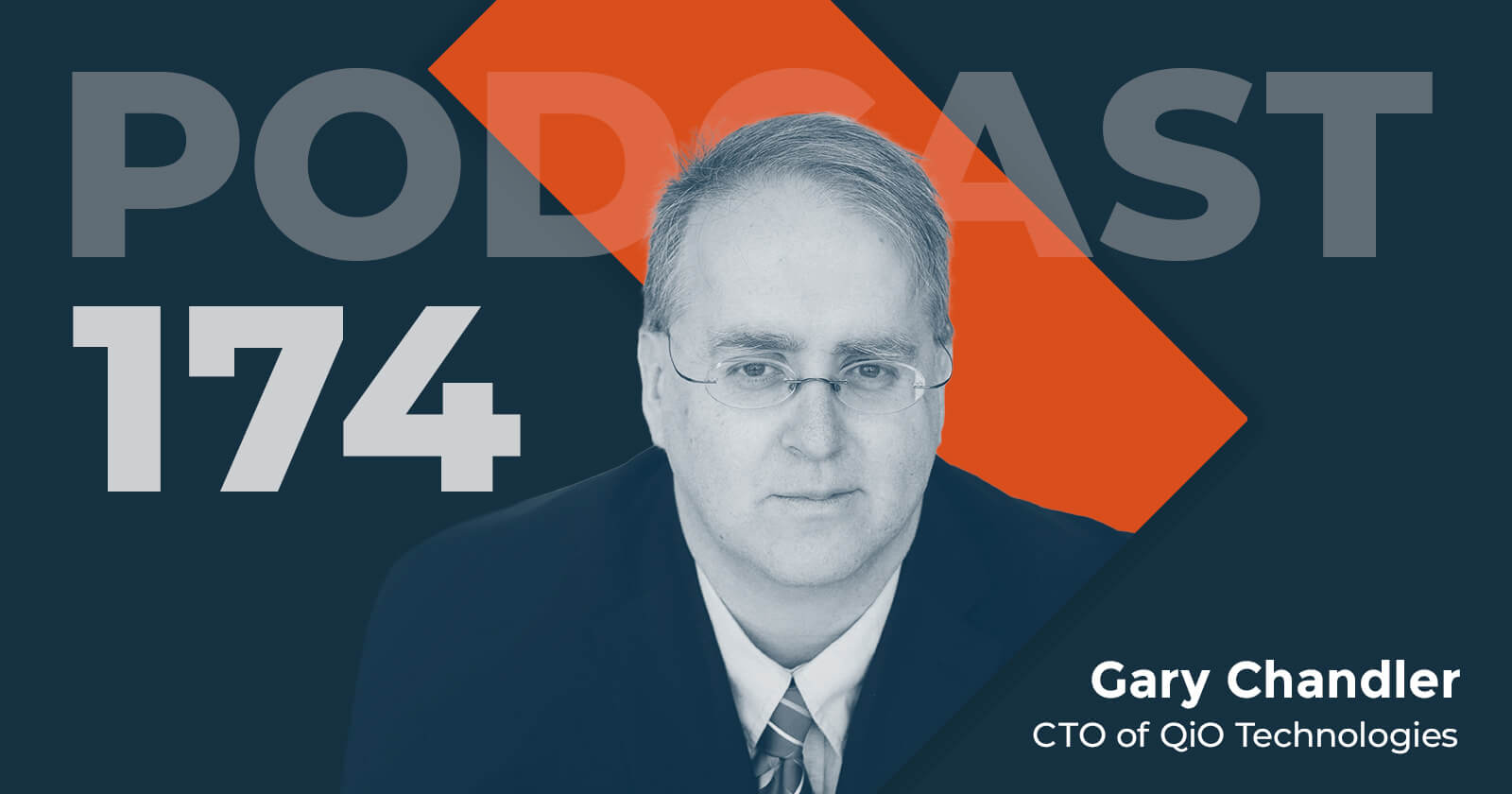
TRANSCRIPT
Ken: This is Ken Forster, executive director at Momenta. Welcome to our Digital Thread podcast produced by, for, and about digital industry leaders. In this series of conversations, we capture insights from the best and brightest minds in the digital industry, including executives, entrepreneurs, advisors, and other thought leaders. We hope you find these podcasts informative, and as always, we welcome your comments and suggestions. Like our team at Momenta, they have in common: they are deep industry operators.
Good day, and welcome to episode #174 of our Momenta Digital Thread podcast series. Today, I'm greatly pleased to have Gary Chandler, Chief Technology Officer of QiO, providing AI solutions to optimize industrial assets. Gary has over 30 years of experience in engineering real-time embedded software control systems for safety-critical applications, predominantly aerospace. Gary spent 25 of those years at Rolls Royce, where he served as Chief Engineer for engine control systems and later as Chief Data Architect and Head of Software for the firm. He spent the recent five years focused on delivering Internet of Things technologies at QiO to large industrials to help them generate value and realize their digital roadmap. Gary, welcome to our Digital Thread podcast today.
[00:01:28]
Gary: Thanks, Ken. I'm a longtime subscriber and fan of the series. It's a real pleasure for me to be joining you today.
[00:01:35]
Ken: Real pleasure to have you. We'll talk in a moment about why this deep fascination with your historical background at Rolls Royce and QiO, but suffice it to say, it's great to have you on the program. I always like to start in terms of one's digital thread. What would you consider to be your digital thread? In other words, what's the one or more thematic threads that define your digital industry journey?
[00:01:59]
Gary: I grew up in the '70s and '80s, in an area on the west coast of Scotland, which was known as Silicon Glen as opposed to Silicon Valley. It was the high-tech industry hub in Scotland. My father was an engineer at IBM, and my eldest brother worked at IBM. From an early age, I was surrounded by developments in digital electronics. I was quite lucky to have access to the very early personal computers of the day, including the IBM PC when it was first manufactured in Greenock in the early 1980s. Those early PCs were targeted at word processing and accounting tasks. I remember my main interest in them was always trying to connect electronics to their IO ports and create some interaction with the real world in some way, even if it was just something as crude as a proximity detector. I'd say that was the beginning of my digital thread, which led me to obtain an electronics degree in '91. While I was studying for a degree, I also worked at IBM during my vacation periods, and the work was interesting. I realized didn't want to design IT systems or office software; I wanted to work with control systems. It helped me realize that I was more interested in robotics and the automation on the manufacturing line than I was in the PCs being made. When I was looking for my first job after graduating, I focused on working with control systems. I was pretty fortunate to land my first job in '91'91, working on engine control systems for Rolls Royce jet engines. I continued to do that for the next 25 years.
[00:03:45]
Ken: It's interesting when many of us talk about working on control systems. It's usually those having to do with the pumps or machine tools in a factory or such. Still, to go right to a mission-critical application like engine control systems which many of us have experienced over our life working correctly in terms of our flights, that's an exciting jump to be able to make. In an age where we're inundated with terms such as 'servitization' or 'x as a service,' let's go back to 1962 when the Bristol Siddeley company filed the trademark for “Power by The Hour.” This was for a complete engine and accessory replacement program billed at a fixed sum per flying hour.' The then-owner of Rolls Royce reinvented the program in the 1980s with the same basic promise but including all services power by the hour. Now, 60 years later, that trademarked “Power by The Hour”, is used to describe x as a service. I've long wanted to interview someone close to this program, and I'm quite pretty that we met. To get some context, perhaps you can take us back to your earliest experiences with this program at Rolls Royce.
[00:05:02]
Gary: I can't take you back to 1962, but I will take you back to the mid to late '90s. That's when I was introduced to the 'power by the hour' program in any meaningful way. By then, the business model had been developing for many years. The basic premise of “Power by The Hour”, or servitization, as you know, is that the product's end-user doesn't ever buy or ever own the product. Instead, they buy the results of using the product. Hence, for the jet engine that was “Power by The Hour”. The most important part of “Power by The Hour,” or servitization, is knowing what price to set for the hourly use of the product. Of course, that depends on a whole host of factors. Primarily, you must predict how the asset will be used and how it will degrade. To maintain the assets, you must determine how, when, and what cost. Once the asset's operational, you must monitor to check the performance according to your expectations. If they're not performing, you're going to have to intervene before you lose your profit margin.
Simply put, you need data to run an effective servitization model. The data driving the power by the hour model for the jet engines was largely generated by the embedded control systems for which I was writing the Software. It was clear that the more information you could derive from the data and the better informed we could be about revenue projections, and this theme around the value of data continued throughout my career. I was constantly wrestling with how to get more data off the engines. You mentioned the 1960s, but from my perspective, the Trent 700 jet engine that entered service on the Airbus A330 in '95 was the first real example of the servitization model as we know it today. The big difference from those early days was that engines before the Trent family of engines could consume several spares equivalent to the original cost of the engine in about eight years. The technology advancements on the Trent family of engines extended from eight years to around 25 years. It changed what happened in the OEM maintenance cycle of maintenance, repair, and overhaul, or MRO services, as we call it. Before that time in the '90s, it was pretty limited to warranty repairs. MRO work in general was largely carried out by technical services divisions of the airlines and dependent MRO providers. The reality was that over the lifetime of the engine, the spares and repairs business could be even more lucrative than the sticker price for selling the products in the first place.
Some independent MRO operators were more intimate with customers in the airlines than the OEMs themselves. When you couple that with the projected loss of spare revenue due to the inherently more reliable engines, and the opportunity to form stronger customer partnerships, it made a lot of sense to deliver power by the hour. That generated a lot of commercial success, being the first to market with that model. Until the competition caught up, there was a bit of fun with marketing at the time. I remember things like, "Why do you pay our competitors when their products break? Pay us when our products work." And today, I still think that would be a great tagline for anyone stealing a march on their competitors with a servitization model. Data was so important to the successful running of the “Power by The Hour”, network. The Rolls Royce in 2004 opened the state-of-the-art operation centers at Derby in the UK. If you think about Mission Control at NASA, you get a picture of what that operation looks like. The Ops Center pulled data in real-time from aircraft engines and maintenance facilities. It allowed them to mobilize resources to service any aircraft anywhere globally.
[00:09:19]
Ken: It's interesting given the level of planning you went through on this or considerations of the various factors, including revenue streams, and then the learnings subsequent. Not only were you first to market with it, but you guys were also the trendsetter. Again, this whole idea of power by the hour exists as a tagline across the industry anytime we talk servitization. We see a lot of industrial OEM's concerns about making that move. They claim their shareholders aren't ready to jump from CapEx to recurring revenue models. Yet you guys already had a lot of experience in this. I'm curious, how did this as a service manifest itself in your daily engineering and operations activities? What do you think was different in how you guys were approaching it versus your competitors who were still catching up at the time?
[00:10:16]
Gary: I think right from my very first experience with “Power by The Hour,” I was quite inspired by it. I probably didn't use the term sustainability much in 1995. I remember many discussions you would have in today's sustainability space. Those discussions started around questions of why customers need to own products to use them. That was a very alien concept in that world where we had been selling big-ticket items and then benefiting from the spare's revenues. It was quite a hard model to move away from, but because we were introducing the advanced technologies and improved reliability, we recognized that those spares and repairs revenues would start to diminish for us, so we had to change how we thought about the whole lifecycle of the product itself. The as a service mentality did permeate through the daily engineering, manifestly challenging us to design the whole lifecycle to sell the high sticker price and think about the impact unscheduled maintenance had on our customers' operations. It made us think about countermeasures we could deploy to minimize any customer disruption. To this day, I still believe servitization is the most eco-friendly business model I've seen. You're right to point out the reticence, and I recognize moving from CapEx to the OPEX, the recurring revenue models. There's a lot of uncertainty with the servitization model, particularly what to charge by the hour to remain profitable through the whole product lifecycle. In the case of the jet engine, you have to set that upfront, and you're going to be running that engine for 25 years. That is a big decision to make, and a lot of uncertainty there. As I said, the point is, many companies make good profits from sales and support of parts after the sale. What is the incentive to change given the uncertainty that you switch to? If you can reduce the uncertainty, if you can effectively take that away using data, you can start to understand incentives across the end-to-end supply chain and make it a win-win for all.
I'll give you a couple of examples and see how that manifests itself. Maybe little extreme examples to show the effect of lack of data or predictability on the servitization model. Suppose you think Rolls Royce had no warning of the Coronavirus pandemic or Icelandic volcanic eruptions, dispersing ash clouds—no prediction because there was no data—the impact of global-scale events on our servitization businesses is very significant. Of course, non-servitization businesses aren't immune to those events either, so I would remind those pointing at these examples as a reason to avoid the servitization model. Regardless of your business model, if your end user's business can't be successful, that will ultimately impact your business. The servitization model differs because it drives innovation in the product company. When the volcanoes erupted and grounded all flights between the US and Europe because of the damage volcanic ash can do to jet engines, Rolls Royce immediately set to work, understanding just how much ash would cause how much damage. They collected data and built a model that meant aviation authorities no longer had to ground flights. Today, with that model, everybody knows how to fly through ash clouds safely. The servitization model incentivized the supply chain to find a solution to minimize disruption and impact on the end-user business. That quickly brings out the subject matter experts wherever they sit in the supply chain. Everyone else wins, too, and rather than sit on the cash in the bank from big sticker price sales while your end-user suffers, everyone rolls up their sleeves, and as Plato said, necessity is the mother of all invention. It's an extreme case, but hopefully, it gives a fair representation of the reality of running a servitization model. I hope this reinforces the importance of data. Data removes uncertainty and drives the business. That's great for all of us in IoT because we're living in a time when IoT technologies allow us to access data on a scale that I could only dream about in my 25 years at Rolls Royce.
[00:14:46]
Ken: What a great example. We often hear the platitudes of upstream suppliers talking about wanting to be a trusted partner to their downstream customers. This is a real-world example of shared risk, shared reward, and what a great way to become a valued supplier and partner. You mentioned data several times, and I know you went on to become Chief Data Architect for Rolls Royce in 2014 and then Head of Software in 2016. Just briefly, what was your mandate at the time? What would you say were some of your key learnings from having held this leadership position at such a prominent industrial OEM?
[00:15:25]
Gary: Three key events happened. I said that the Trent 700 went into service in '95. Rolls Royce formed the dedicated analytics team in '99 to help run that servitization model. Then the operation center opened in 2004, a NASA-like Operation Center, and to borrow a phrase I've heard you use, Ken, connect, collect, and correct. If you think about what we had back then, the engine controls group I was with provided the connecting piece to the data on the engine. The analytics group was responsible for collecting that data from all the engines across the global network and then providing that into the analysis in the ops center that could then do the correct piece taking corrective actions needed to make sure that the whole power by the hour network was delivering what customers needed.
We weren't familiar with the Industry 4.0 terms at the time. But in 2004, we were essentially operating with today's technologies that would look like the Cloud and connected Edge model. If I then fast forward to 2013, I got a call to go and spend some time reviewing the investments and partnerships GE had been making in the Cloud a couple of years before GE Predix was even a thing. It became clear rather quickly that some of the technology and cost challenges we had around collecting and analyzing big data sets were undergoing rapid change. That's what led to creating the position of Chief Data Architect. I was tasked with aligning the chain of data from the engines right through to the ops center across the different groups that had just sprung up across the company over the previous ten or so years. Then in aligning those groups I set about exploiting the rapid developments in IoT technology, which led to the formation of a joint group where we put the controls engineers and the data engineers together. We rebranded that as controls and data services. What I take away from that is 2013 to 2015 was a hugely exciting time for me. You can imagine the access very generously offered to me by the big tech providers Microsoft, AWS, Google, etc. Also, startups gave their eagerness to work with aerospace and with Rolls Royce.
When I saw the potential of the IoT technologies to transform the day-to-day operations, I was hooked—connected equipment, processing power, compute costs, access to huge data sets. Suddenly, they all seemed as though they were in touching distance. By the late 90s, if I take you back and paint a picture, it's that I was pushing the equivalent of one standard letter or only one piece of paper worth of data from each aircraft each day. As I left Rolls Royce, the last engine control system we deployed I could push enough data from one engine in one hour to generate a stack of paper over 20 stories high. The key learnings for me along that journey from data-poor in the 90s to data-rich in 2016 was consistently: How do you make the data you had available at the time work as hard as possible for you? And keep removing the next level of uncertainty from the operations. As you said, I was then asked to go and head up Software. Primarily, that was to drive the balance between opening up the volume of data we could pull off the engine and the analytics we could push to the edge and run on the engine itself.
[00:19:04]
Ken: Talk about leaving on a high, and I say leaving because we know that in 2017, you came over to QiO. What inspired you to make a jump to a pure software company from the work you had done prior?
[00:19:18]
Gary: It was difficult, but I remember sitting at my desk in Rolls Royce in mid-2016 and opening a very posh-looking envelope that contained an invitation to my 25 years long service award dinner. I couldn't believe that 25 years had passed since I graduated. I realized then that I was at a major career junction. I could stay where I was and be very comfortable. Given what I had had my eyes open to in the IoT world as I looked outside Rolls Royce when I was building the IoT strategy, I had developed this concern that access to the power of AI in industrials might get locked into a privileged few. I wanted to try and demystify AI and make it more accessible to industrials who couldn't necessarily afford to employ the sort of dedicated analytics teams that we had at Rolls Royce. Between 2013 & 2015, as I said, I must have looked at over 50 IoT companies, and QiO was the standout company. After getting my envelope in mid-2016, I rather cheekily called up one of the co-founders of QiO, Baz Khuti, and asked if he had room for one more. As you said, QiO was a pure software company, and all my working life, I've worked with software and hardware. That's still my passion, but the attraction of a pure software company is around the pace and agility that comes from decoupling yourself from designing, developing, and building the hardware itself in which the software must run. And today, there are plenty of excellent Edge solutions available on the market to host our software solutions along with our Cloud solutions. That's a great model for us.
[00:20:59]
Ken: We featured Baz Khuti on one of our earlier podcasts, and I tell you, they describe people based on network density, and he's probably a bit of a dark hole in that regard about the densest network I've ever seen in terms of an individual. He has been great in terms of connecting us with people. Now QiO delivers an AI-augmented foresight suite of solutions to accelerate digital transformation, revenue growth, and sustainability for the global industrial sector. It's a mouthful, quoted directly from your website. Practically, what does that mean?
[00:21:36]
Gary: It probably means we need to rewrite, that phrase, but yes. QiO solutions essentially act as a coach and a mentor to our clients to make them operate at their best every day. We know industrial operations have good days and bad days. And sometimes, we don't know whether we had a good or a bad day until analyses are run a few days or weeks down the line. What we want to do is accelerate that digital transformation and help industrials make better decisions faster. Our AI recommends to operators the changes and interventions they need to make in real-time to plant equipment and assets to keep the system optimized for energy efficiency and to be the best version of themselves that they can be today. It's quite difficult to know if you're truly operating at your best right now and ensuring that everyone across the organization is following the right recommendations. I'll give you just a couple of examples. If you consider a quick question that most industrial leaders will know the answer to, it's how much product did you make yesterday, and how much energy was used? Most industrial leaders will quickly access those metrics, which shows they have data and can access the information to answer the question.
But then if you ask, do you know if that was a good day or a bad day? That's a little bit harder to answer. Many folks wouldn't necessarily have that level of knowledge at that time. You can then make the question even harder and say well, "How did yesterday compare to your best day, and why? What instructions do you wish you had issued to make it a good day?" It's getting tough to answer because you're now getting into the realms of wisdom. Were you wise enough at 9 am yesterday to tell everybody what they should do so that the business had a good day? And really, what we've done at QiO is build the application that gets you straight to the wisdom level automatically. As I said before, there are teams of data scientists that could help you get to that wisdom. We want industrials to have quick and easy access to this level of AI. They absolutely should, and they absolutely can. Sometimes, it feels like magic when we try to explain it, but it's not. I'll give a last simple example to illustrate it. If you take a really simple system that most of us, think that we're subject matter experts, and if you take a car, most people understand something about how to drive it to achieve good fuel efficiency. They can probably tell you whether they drove efficiently or inefficiently on their last journey. They intuitively think they know. They may think they know what sort of cost savings there could have been if they had chosen to drive a little more efficiently. They feel they've got a good inherent knowledge of how their car works, understand the outcome they achieved, and understand what it costs. Well, same for the industrial leaders managing plants and assets. If you imagine the front tire on the driver side goes a little flat, it loses two PSI of air pressure. Probably, most of us would not have the knowledge to understand the impact that two PSI pressure drop was having on the efficiency and cost of running the car. For sure, we know the efficiency would be down, but even if we had the data and the information that there was a two PSI pressure drop, we wouldn't know the cost impact. That is trivial for an AI model of that car to work out; an AI model can easily infer what impact the two PSI pressure drop has had on the system as a whole and can therefore associate a cost with it. It can deliver us that knowledge.
Let's now take it to the wisdom level and say you've got three options as to what you might want to do, given that you've lost some tire pressure. You want to know if it would be wise just to carry on driving around for two or three days until the next time you go to the gas station and fill up, and then you can top up the air in the tire. What's the cost? What's the risk? Should you drive to the gas station straight away and just pump the air into the tire or should you drive straight to the repair shop and get a new tire right now? When you break it down like this, you can see that the AI can issue you with a recommendation; option one, two, or three. Of course, you can drill down into the tire pressure data and compare that with previous journeys, etc. But right now, just do this. Follow these recommendations, and you'll get the best outcome and the circumstances. As I said, it's a simple example. It's still difficult to make the right call even if you knew the tire pressure had dropped to two PSI, and most of us wouldn't even be aware of that. We're spending money on fuel that we don't need to spend. We're pumping more CO2 into the atmosphere and weren't even aware of it. Now, project that onto industrial operators. They've been asked to try and make those calls every day from within much more complex systems with all different sorts of assets changing their behaviors as they heat up, cool down, speed up, speed down, etc.
Our AI models recommend the best outcome, do this now. If you take that onto the sustainability piece, we feel strongly that if you wait until the end of the quarter, or even the end of the month to analyze and act, it's going to be too late. You'll be off your net-zero tracking targets, and you'll be out of time to get back on track. What we want industrials to do is to make better decisions every day, every hour, every 15 minutes. That's what will lead to continuous systemic optimization, and that's about becoming the best version of yourself that you can be today, then going on and improving and becoming the best in class. I see a lot of great IoT companies out there working at the data and the information layers. My passion is to use AI to generate wisdom from a customer's data and make this more available to everyone and help accelerate that digital growth, helping companies achieve their sustainability goals and save money. That's our mission.
[00:27:40]
Ken: I think while you are Chief Technology Officer, you might want to don the Chief Marketing Officer hat. I'm glad I asked you this question of what it practically meant and perhaps get to the industrial wisdom level or different variants of it. That was helpful. I think the industry would refer to this as prescriptive analytics. Typically, the descriptive, predictive, and then prescriptive hierarchy gives the options to consider what you should do. What are the trade-offs going to be as you make these decisions? Often, we think about it in an ag-tech sense, instructing the farmer of options in terms of planting, harvesting, fertilizing, watering, and things like that every single day. Recipe management if you will. Can you share some of your key use cases and wins?
[00:28:32]
Gary: Yes, sure. I think probably since 2017 it's fair to say we took more time than we would have liked to truly find our focus and some of our early use cases and money-spinners, they were great solutions, but we're off our True North. In the past 12 months, we've had a new CEO, and he's brought a laser focus in terms of a true software product company focusing on helping industrials. That's around optimizing operation of the assets, monitoring the health of the assets, and servicing the assets as efficiently and effectively as possible. I'll focus on use cases that came through from those early days. And it won’t come as a big surprise given our conversation at Rolls Royce that they use our software to help manage their network of over 80 maintenance shops around the globe servicing more than 6000 of their business jet engines. That's such a great reference for us as a business-critical application running in the Cloud and with our marquee client since 2017. In the energy-intensive industry, we've had some early successes in energy optimization and carbon reduction, such as glass and steel manufacturing. We can typically deliver savings in the range of 5 to 15% straight out of the box. I also want to mention that we are privileged to partner with BT and provide their customers with our Foresight Sustainability Suite. In terms of being a pure software company, partnerships like BT are invaluable to help us achieve wide reach with our Software, coupled with BT's expertise running their client factories' IT networks, data centers, and security. We've got a fantastic Edge-compute offering. As an embedded software engineer, I'm excited about what the Edge roadmap will deliver for customers, and I really can't wait to roll those features out.
[00:30:24]
Ken: How do you know when an organization is ready to adopt your solution? What you're providing is advanced. What are some of the best practices you've seen in clients realizing the potential value of that solution?
[00:30:38]
Gary: In some respects, it is advanced. But in other respects, it is very accessible. You're right in saying that we need C-level sponsorship because of what our product can do to the business. It's great for us to find leaders who recognize that there could be more insights in their data than they've been able to extract. Those leaders are humble and ambitious enough to realize that they are not operating at their best every day. That's a great opportunity for us to work together and deliver some value. Connecting to the data sources is still the biggest friction point to adoption. Making sure that you don't burden the client to solve that connect problem for themselves, I would call out probably as one of the most important best practices.
[00:31:32]
Ken: Finally, in closing I'm curious, Gary. How do you find your inspiration?
[00:31:37]
Gary: I credit one book to changing the course of my engineering career for the better, and that's "Lean Software Development" by Mary Poppendieck, published in 2003. I would encourage anyone in engineering to read it and not be put off by the word 'software' in the title. After years of trying and failing to transition the benefits of leading manufacturing into the office and engineering environment, that's the book that changed me forever. It has been read many times and is the permanent fixture on my bedside cabinet. Lastly, my greatest inspiration has always been talking to customers and colleagues. I've been fortunate at both Rolls Royce and QiO to work with some super smart people and customers. I'm constantly reminded every day that I'm still learning.
[00:32:21]
Ken: Sounds like a lifelong ambition and vision, which is a great one to have. Gary, thank you for sharing this time and insights with us today.
[00:32:30]
Gary: Thanks. It has been such a pleasure for me and a real honor to have been invited, so thank you.
[00:32:35]
Ken: Absolutely. I appreciate the fact one, you're a longtime listener. Two, you quoted me at least once in there. You're what I call a critical listener. And three, I think you brought a lot of value into a conversation that many of us often think of as a new-age problem and a new-age solution. It's always helpful to understand how major leaders in the industry have approached it. It's great that QiO is taking these learnings and making them accessible to the longtail of companies that are out there. We look forward to hearing lots of great things in terms of QiO. This has been Gary Chandler, Chief Technology Officer of QiO, the company providing AI solutions to optimize industrial assets. Thank you for listening, and please join us next week for the next episode of our Digital Thread Podcast series. Thank you and have a great day.
You've been listening to the Momenta Digital Thread podcast series. We hope you've enjoyed the discussion. As always, we welcome your comments and suggestions. Please check our website at momenta.one for archived versions of podcasts as well as resources to help with your digital industry journey.
Thank you for listening.
[The End]
Connect With Gary Chandler via LinkedIn
Gary's Inspiration Comes From...
"Lean Software Development," according to Gary, is the book that changed his life forever. Mary and Tom Poppendieck, the authors, take lean industrial practices to a new level by explaining how to apply them directly to software development. Additionally, he notes that his greatest source of motivation is conversing with customers and coworkers; they remind him that each day presents a new opportunity for learning.
About QiO:
Founded in 2015, QiO Technologies is the only Industry 4.0 company built by industrial engineers with the goal of accelerating progress towards a zero-carbon economy.
QiO uses AI for pattern recognition to zero in on raw data and deliver prescriptive and predictive actionable insights for data-driven decision making. Engineered for rapid deployment and industrial scale ROI, QiO built their solutions with an open-source philosophy with no legacy encumbrance on any cloud or software architecture nor prebuilt templates and that enables them to adapt to new sectors quickly.
Learn more at https://qio.io/.