Michael Risse
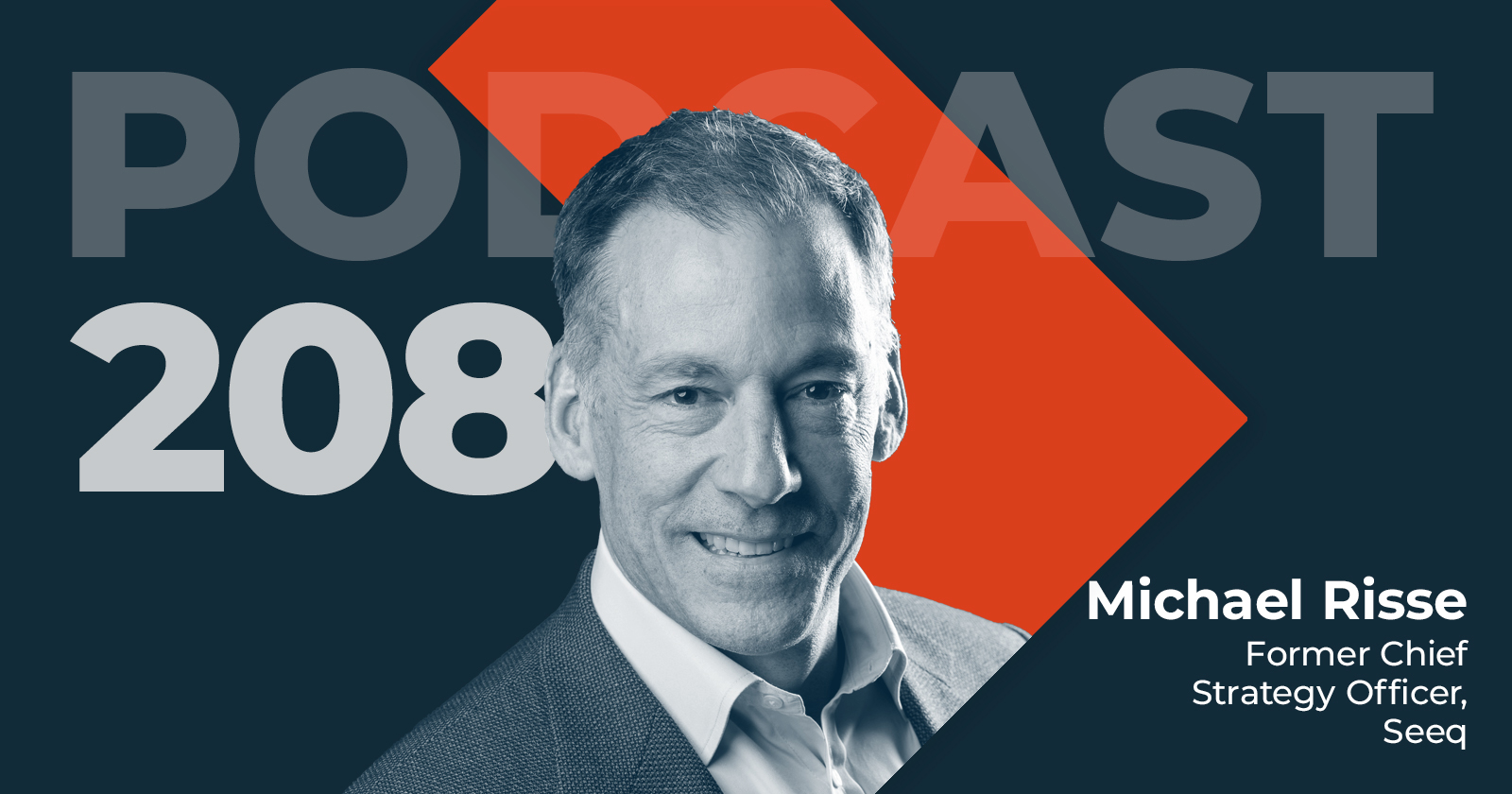
TRANSCRIPT
Ken: Good day, and welcome to episode 208 of our Momenta Digital Thread podcast series. Today, I'm pleased to bring back Michael Risse, a founding partner at Seeq Corporation, transforming industrial process data into actionable intelligence. His work at Seeq is built on 25 years of experience spanning traditional software businesses, startups, and large enterprise management roles within Microsoft. Michael joined us for episode 198 earlier this year, during which we started deep diving into Cloud vendors and operational technology. By popular request, and honestly, I got much feedback from our listening audience on this one, we brought him back in a 'rest-of-the-story' episode. Michael, welcome back to our Momenta Digital Thread podcast.
[00:01:26]
Michael: Thank you very much. It's great to be back.
[00:01:28]
Ken: Again, by popular demand. You've left a nice cliffhanger hanging out there and certainly hit a thread of interest among our listeners, so let's dive right in. First question, what do you see as the evolving role of Cloud platform companies in OT? Let me be clear, in our last episode. I refer to these as the hyperscalers, which is the popular term between you and Andrew Obin at Bank of America. You convinced me that we refer to them as Cloud platform companies or vendors. That's what I'm referring to when I talk about the evolving role of such companies in OT.
[00:02:03]
Michael: Got it. Let me start by paraphrasing Winston Churchill: where are we in this market? We're at the end of the beginning, right? If you think about the start of the Cloud, whether it's AWS or Azure, you're talking roughly ten years from 2000 when they announced it, or when they shipped it – so 2004, 2006, 2008, 2010… That’s the first decade-ish in the Cloud business or in the Cloud world. What happened is that Microsoft and Azure, and AWS, both started with basic workloads and then kept moving up the stack and moving to the next workload and the next workload.
Meanwhile, for a while – let's see – in 2014, 2016, it seemed like manufacturing cloud workloads were going to be owned by automation vendors like GE with Predix. You may recall Jeff Immelt saying that GE would be a top 10 software company and, obviously, Siemens with MindSphere and other initiatives. Both through had challenges in being software companies and enabling that experience.
Meanwhile there was growing Cloud vendor enthusiasm for aggregating manufacturing data on the Cloud with 2018 as a tipping point. That fall there were articles in The Economist, the Financial Times, and The Wall Street Journal, talking about Microsoft and AWS focusing on oil and gas in particular but on manufacturing in general. That was the start of their new investments, services, and product offerings, and you get to the point where they are today.
Look at CERA Week, the big multinational executive oil and gas conference: Microsoft and AWS are both big sponsors. You look at ADIPEC, Abu Dhabi International Petroleum Exposition: again, Microsoft and AWS are big sponsors.
You've gone from where stage one where Microsoft and AWS had other interests. In stage two, GE MindSphere and others tried to lead the effort on manufacturing in the Cloud. Finally, in stage three here come AWS and Microsoft, and the momentum is there, the investments they are making, and it's becoming more prevalent that every customer has a Cloud roadmap. It's just part of the thinking, and as I said, it's the end of the beginning, and now we're moving into production or actual implementations of these ideas.
[00:04:31]
Ken: It's interesting; you quantify it as the end of the beginning. Of course, the other side of it is the beginning of the end, and I'll quote somebody you and I like; that's certainly Andrew Obin of BofA, the one who covers all the industrialists. In a report on Rockwell Automation, he recently wrote, particularly, "The challenge from Cloud platform companies appears to have diminished recently, but given these companies' financial capabilities, we think are still worth watching." It's interesting because Andrew has been, even at the beginning of your report, which we talked about in the last podcast, has been quite bullish on the Cloud platform. For him to take, a neutral position on this is quite interesting. Thus, the comment about the beginning of the end. What do you make of his perspective?
[00:05:19]
Michael: Two thoughts on that. First, Andrew said that given their financial capabilities you don't want to count them out. Let's take a super specific example.
Microsoft announced Time Series Insight, or TSI, in the spring of 2017. TSI was a time series data storage and trending service; it was the endpoint for their IoT offerings which the data would flow into. It had an interesting concept around enabling both hot and cold storage. You had hot data that was more expensive and recent, and then cold data that would be cheaper and could be stored more efficiently. The TSI value proposition did not work out and the service ended last spring, so it was out for about five years. They've refocused now on ADX (Azure Data Explorer) as their primary store for time series data and looking more to partners to provide the analytics on top. Now, that's what you can do if you're an AWS or Microsoft. They can take a five-year view figuring out they didn't have it right and restarting and saying, "Nope, we're going to go in this other direction." That's what those financial capabilities enable for AWS and Azure so I wouldn't count them out. The other interesting thing is that I don’t know if the world has figured it out or has the right answer for how this will work.
Second, I'm not the first person to say this, but what happens to the Purdue model? Is it the end of the Purdue model? What that means is what used to be an on-premises five-layer network and security architecture; well, the top of that Purdue model is moving to the Cloud. The enterprise applications have already moved but of manufacturing what's on-prem, what's in the Cloud, and what workloads will go to the Cloud, that still has to be sorted out.
I'm a strong believer that manufacturing data, as we discussed, will move to the Cloud. Analytics applications and machine learning analytics - those are going to be run in the Cloud. APM - Asset Performance Management - is an application category that can and should be run in the Cloud for integration with other systems and for centralization purposes. And there's some data hub or asset model that should go to the Cloud.
But the details of how that works and which service you're using- that's not known yet. As that gets clearer, I think that'll make it easier for the automation direction of the Cloud platforms to say, "Okay, these are the services either we need to build or partner for."
But right now, that is unclear - again, we're at the end of the beginning. That's a common conversation, but it hasn't landed yet in architecture, guidelines, blueprints, etc. Given their financial capability, we've got 5, 10 years to go in this transition, and the Cloud vendors will be there. As the services sort themselves out, it'll get clearer about where they want to invest and how they want to accomplish it.
[00:08:13]
Ken: I think, certainly, the triggers for Andrew's comments, and what has happened subsequent to your and my conversation earlier this year, is that Microsoft laid off a large part of their "industrial or manufacturing team." For the third time in their history, GCP blew out their whole team. They've had a dream team twice that I'm aware of, and the whole team left, literally. It was like, you couldn’t login in the morning. "Oh, by the way, we cut your accounts across the board." We're hearing this from senior people, so it was a hard cut. AWS has at least maintained, if not continued, its trajectory. Is this just a two-horse race now? Is it just Microsoft and AWS going after this? Or do you still see other potential Cloud vendors who might make some traction here?
[00:09:08]
Michael: I've been emphatic and realize I need to put some context around my opinion, or somebody will get annoyed. But I've been emphatic that it's only a two-horse race: AWS and Azure. It absolutely is. Only two companies on the planet can put two data centers in every interesting geography, which is what is required for data regulations and backup within a country. That said, the audience for the two data center requirements will be multinationals as they cross country borders. For example, if you're in China, then Alibaba has a conversation, but not beyond that.
But as soon as you get to multinational enterprise organizations their checklist is “Are you where I operate? Do you have two centers in countries where I operate? Do you support the data regulations associated with each country?” When you combine those three requirements, the list of Cloud vendors gets very short.
The other thing is more than just the data and the physical infrastructure of these Cloud platforms. I call it the 'dark arts' of the Cloud platforms. Microsoft, for example, has something called MACC, the Microsoft Azure Commitment to Consume. AWS has something called EDP, which is the Enterprise Discount Program. Those programs offer discounts if customers commit to certain spending limits on these platforms. Thus, customers want to spend on those platforms because that's where they’ll get the discounts, but only if they reach a certain spending level. This means you've got senior IT and procurement involved, saying, "Look, I want to hit these spend levels so I can get my discount, and we can pay less for our Cloud services." What does that have to do with manufacturing stuff? Well, very little, except that IT now has its nose and its self-interest in manufacturing software purchase decisions.
IT in general also has its nose in it because they want to have a cogent strategy for their Cloud platform decisions, which means more things on one Cloud, or on the same Cloud, and a single roadmap for that Cloud.
IT security, as another example, the folks who oversee compliance and things like that, have an interest in the Cloud discussion. Their ability to checklist requirements that don't have to do with how wonderful the manufacturing software is but have to do with the ability of vendors to get through IT process - the procurement, the security, and the strategy checkpoints. These requirements, again lend themselves very well to Microsoft or AWS, as opposed to other companies that aren’t going to be able to offer discounts or aren’t going to have senior relationships with IT, the CIO, if not the CEO, of the company. You look at companies and ask, "Who's your most strategic vendor?" The answer is AWS and Azure. For these reasons, it's a steep cliff from AWS and Azure down to whoever's in third place.
The last thought is what happens in real, open software markets is software markets consolidate; that's what happens. You get down to one or maybe a couple of options. For example, you’ve got SAP and not SAP (i.e., whoever is in 2nd place); you’ve got Google and not Google; you've got Salesforce and not Salesforce; you've got Microsoft Office and not Microsoft Office. This is what happens due to the natural consolidation from economies of scale and network effect, the incentives in IT, and the investment requirements to build and run these data centers.
That's why I'm emphatic it’s an AWS and Azure world. Again, I'll leave the caveats in that this is a multinational company perspective. There could be local organizations within one country or, as I said, Alibaba in China. It will be the exceptions that prove the rule.
[00:12:42]
Ken: This has provided a good context of the landscape, the vendors, etc. Let's now notch down and talk about how OT companies should think about and plan around the Cloud, which gets to the point that we talked about last time in terms of your prediction that they will be.
[00:13:01]
Michael: The interesting thing is how well the enthusiasms align. The Cloud platforms are enthusiastic about manufacturing workloads, data, and business opportunities.
Meanwhile the customers, the companies, the actual manufacturing firms, they're driving just as hard towards Cloud-based solutions for their challenges. Where is machine learning going to run? Where are data scientists working in Where do you want the manufacturing data with machine learning and the data scientists? In the Cloud, right?
What are you trying to accomplish? Better efficiency, optimization, and sustainability by looking across our plants, then the data from those individual plants should be rolled up and accessible. Where? In the Cloud.
What’s a modern way of helping to attract younger employees, when you create a center of excellence in a major city where it's easier for them to live and work. You bring or enable the data access for these experts in a particular asset, class, or role to work on the data, regardless of where the plant is. The center of excellence motion, it's something I've seen in several companies. What's the best way to architect that? Oh, that's going to be in the Cloud.
How do you get smart about your Cloud data or your manufacturing data? You contextualize it; you put it in the context of other business applications and outcomes. Where are those applications? Oh, those are running in the Cloud.
You start going down this list of what manufacturing organizations want, hiring new employees, tapping machine learning, looking across plants - all of those things point to the same place, which is data and workloads in the Cloud. Customers are moving to the Cloud as much as the Cloud platforms are interested in moving manufacturing data to the Cloud.
There's enthusiasm on both sides, and that's why, again, back to Churchill, this is the end of the beginning. These things align and start; we're not in the beginning anymore. We're moving into the alignment of these two perspectives (Cloud vendors and Cloud customers) into execution.
[00:15:07]
Ken: What are some of the best practices you've seen around Cloud deployment for OT companies?
[00:15:14]
Michael: Obviously- maybe not obviously, but certainly what you want to avoid is analysis paralysis. As I said, this decoupling or Purdue 2.0 has not been sorted out. There are some amazing conversations among solution architects, vendors, and customers figuring out what is the balancing act between on-prem and Cloud. But it hasn't sorted itself out, and the challenge from that is you get into analysis paralysis that, okay, I want to get everything right and only then am I going to enable the value.
Instead, companies need to turn that around so the value can happen sooner. You don't want to architect first and then find out "Oh, oops. That actually wasn't the most important piece."
What are the priorities? First, getting fast results so you know what it is that you're designing for would be one.
The second one would be recognizing there's no value in the data itself, particularly time series data, so a bucket of time series data is a bucket of time series data. There's no value in the data. It's unlike relational data, where you have transactions, names, and important information. In time series data, you've got zeros and ones until you contextualize it.
Finally, enabling value by various audiences on the data is super important. It's not to store the data; that's not a benefit. How do you enable value among the audiences that need insights?
I think about certain data scientists and machine learning; how can they get to data and get value? That's a very different data access pattern than your process engineers that may be looking at ad hoc type scenarios, or your managers or monitoring applications, that's more of a streaming context of getting information out and turning it into value.
And you may want something structured or semi-structured so that the data can work for your business analysts using Power BI and Tableau as their products.
You have to think about these user categories and the data access patterns they require to get the possible value out of this storage system. Getting the value out and avoiding analysis paralysis because you don't know what you'll need and thinking about the value at the endpoint.
So support for those different audiences, their data access patterns, and each of the tool sets is high on my list. I say that partly because Ken, you and I have seen many architecture diagrams where sensors and data sources are on the left side. In the middle, there's this big intestinal-looking thing of wonderfulness: the data lake, the data hub, the data something, whatever. And over on the far-right side, in a small font, is the users of this data.
This diagram is not drawn to proportion; the value is on the far-right side where the data scientists, business analysts, and process engineers are; the monitoring applications and the managers get value. I don't know how you design that architecture with them in the middle. Still, the data should serve their use cases, and access and value should certainly be central or more central to those discussions.
[00:18:20]
Ken: Certainly agreed. Those users probably have a heightened expectation with the hype of generative AI; large language models call it ChatGPT. If I can find the best pizza recipe for the local region, why can't I use it to analyze my process? I'm not kidding; it's venture capitalist. We are getting inundated with companies popping up to literally to solve for this. But where we're seeing the greatest impact is back into, as you describe it, the intestinal block in the middle, especially data ops, context, setting, etc. What do you see in terms of OT based on this interest in generative AI? Where do you see that going?
[00:19:09]
Michael: Wow, what an interesting six months it's been. While we thought the internet took off fast, the ChatGPT interest has been incredible. So much awareness and access - at least in the early days, you couldn't use the internet as easily to write your term papers as a college or high school student.
But the issue I've seen previously, and still see, is - and on this, I'm borrowing again - before, I had Winston Churchill; now, I will borrow from Martin, the CEO of Raven AI, one of Momenta’s investments. We've had some great discussions about AI in the manufacturing context.
What's the Tesla moment? Have we reached the Tesla moment for AI in manufacturing?
Martin and I agree. No, we have not. We can get into a car with a stranger. That's an Uber moment. But when you get into a self-driven car, that's a Tesla moment. Like right now, when there's a Tesla error and a crash based on the failure of the driving system, it's national news. That's one car and its passengers. As interesting as this innovation is, can it really be trusted? In a manufacturing context where the safety of the employees is the number one priority then what's the balancing act with AI?
What you said was interesting in that it's not at the plant operation level but at getting data right for use and access. But when do we get to the Tesla moment and trust that it's still interesting? Machine learning's okay; that's one thing. Those are algorithms that people understand or are comfortable with, but how do you get to that Tesla moment where you don't understand it, but you still trust it, and you're going to make decisions on it. Whoosh!
We could have a great conference for several days and get perspectives on what it will take and how that will work. In the meantime, the hype is just going to keep going. Whether you're a venture capitalist, whether you're the parent of a student, or whether you're a senior executive, it's like, "How do we use this? How do we use this? How do we use this?"
That approach runs up against a brick wall of “Look, I'm not trusting the safety of my employees and colleagues to something I don't understand, period. Boom. Get it worked out.”
[00:21:28]
Ken: Look, if nothing else, we've talked about this a lot internally in terms of the investment thesis; it is amplifying the simple fact that OT data is relatively unclean, i.e., your context is not always set. A lot of it to your point about time series data needs to be more relevant in terms of the large language model-style learning capabilities. But we're seeing a general uptick in every company we've invested in that operates in the "DataOps space." All of a sudden, much interest is coming in because that realization is hitting pretty hard, and all of a sudden, these companies are becoming interesting properties. It goes back to your analogy about the people on the left there. As those people discover new tools and capabilities, and ChatGPT certainly would qualify there, it forces more modernization or acceleration of the rest of that sequence from sensors. That's what we're seeing right now; interesting enough. Look, there's been a great conversation. The last time we talked, you mentioned at the end that you had just left Seeq. What's transpired since then, and what are you up to?
[00:22:37]
Michael: Obviously, I am still very interested in the space where manufacturing meets software meets Cloud. Again, this transition is the end of the beginning, particularly thinking about the early manufacturing workloads I mentioned, like time series data storage, analytics, APM as an application space, and then the data hub data layer. But what is going to transfer, how it's going to transfer, who are the interesting startups in that transition to the Cloud- that's been my primary focus. It's just incredible to see the momentum. Interesting startups, interesting places.
[00:23:10]
Ken: I hear from across our portfolio, and generally in the ecosystem, that you are having a broad impact across many companies. As you mentioned at Raven AI, Martin is certainly a proponent of yours. I am a raving fan. If you're doing that across the board, it sounds like you're up to great things, and I'm glad to count you as part of our Momenta ecosystem in that regard.
[00:23:36]
Michael: Well, it's awesome to hear, Ken. I appreciate the opportunity to talk with you and the questions and conversations from here. It always takes my thinking to another level, so very much appreciated.
[00:23:47]
Ken: I also appreciate you taking the time again to do this deep dive with us, and I certainly look forward to the next conversation. This has been Michael Reese, former Chief Strategy Officer at Seeq. Thank you for listening, and please join us for the next episode of our Digital Thread podcast series. We wish you a great day; take care. You've been listening to the Momenta Digital Thread podcast series. We hope you've enjoyed the discussion, and as always, we welcome your comments and suggestions. Please check our website at momenta.one for archived versions of podcasts, as well as resources to help with your digital industry journey. Thank you for listening.
[The End]
Connect with Michael Risse
What inspires me?
As an evangelist for analytics, I am inspired by stories of data and insights in any field. Great reads include books about the use of data in sports (Moneyball, Michael Lewis), dating (Dataclysm, Christian Rudder), and populations (Everyone Lies, Seth Stephens-Davidowitz). For something shorter, the best magazine articles that describe the intersection of data volumes, algorithms, and insights are “The Petabyte Age” in Wired (June 23, 2008) and “The Perfect Milk Machine” in The Atlantic (May 1, 2012). Although both are over ten years old, they are approachable to anyone and clearly articulate the benefits and opportunities in analytics.
About Seeq
Founded in 2013, Seeq publishes software applications for manufacturing organizations to find and share insights rapidly. Oil & gas, pharmaceutical, specialty chemical, utility, renewable energy, and numerous other vertical industries rely on Seeq to improve production outcomes, including yield, margins, quality, and safety. Headquartered in Seattle, Seeq is a privately held virtual company with employees across the United States and sales representation in Asia, Canada, Europe, and South America. To learn more about Seeq, visit www.seeq.com.