Ramakrishna Nanjundaiah
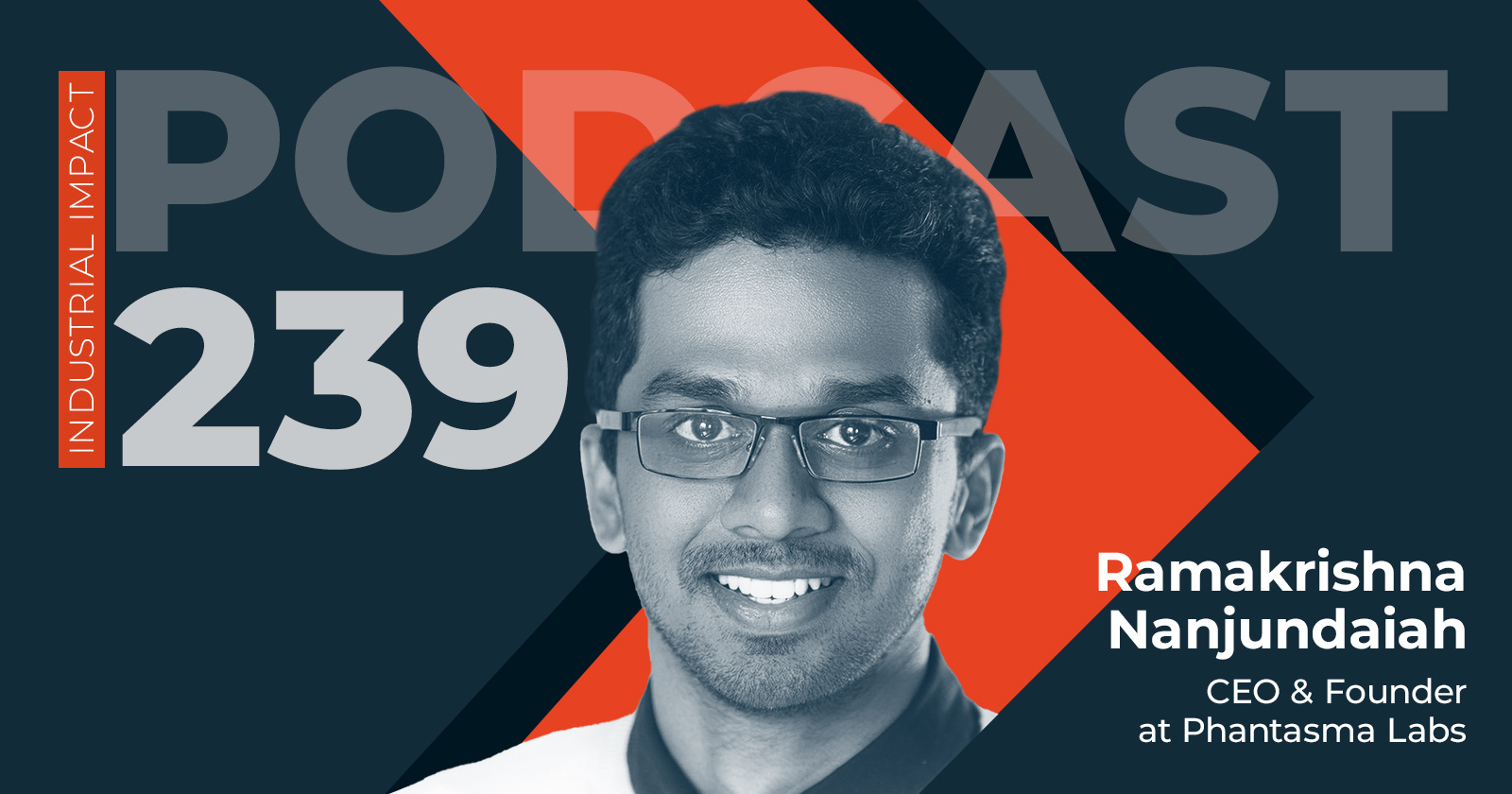
TRANSCRIPT
Ken: Good day, and welcome to episode 239 of our Momenta Industrial Impact Podcast. I'm pleased to introduce Ramakrishna Nanjundaiah, CEO and co-founder of Phantasma Labs, a company transforming production planning and manufacturing with its reinforcement learning AI technology. Phantasma is our newest investment from our industrial impact fund.
Rama is a computational mechanics and AI-driven innovation leader with significant experience across the automotive, aerospace, and architectural industries. At MAN Diesel & Turbo, Rama developed the first AI-driven generative models for gas turbine blade profiles. At SL Rasch, he created one of the world's most extensive pedestrian simulations, modeling the movement of 2 million people in Mecca. His expertise includes creating simulations for Airbus to improve aircraft thermal comfort and evaluating mega structures in Qatar and Saudi Arabia. Rama holds a master's degree from the Technical University of Munich.
Rama, welcome to our Industrial Impact Podcast.
[00:01:48]
Rama: Great, Ken. Thank you for having me on the podcast.
[00:01:51]
Ken: We're very proud to be an investor in you guys. What I appreciate about your team's DNA is the depth of understanding in large-scale reinforcement learning applications. You've got a great story to tell in terms of your origin. But first, let's start with your personal origin story. We always like to ask: what would you consider your digital thread? In other words, what are the defining experiences that brought you to this point?
[00:02:20]
Rama: Sure, I like that question because it highlights my transition from a classical mechanical engineer to building AI and simulation models for enterprises. My digital thread lies in scientific computing, which encapsulates both classical computing paradigms and the new ones emerging with AI technologies.
New AI models are, in some ways, computational models of the human brain. When I graduated in India with my degree in mechanical engineering, I thought I'd spend a large part of my life solving equations related to mechanics and fluids. But that changed drastically when I did my master's in scientific computing at the Technical University of Munich. That was my first defining point in transitioning into this digital thread rooted in scientific computing.
I understood how one could leverage modern computing paradigms to solve real-world problems there. This understanding was further reinforced through a series of exciting roles in the field, where I had the opportunity to build some of the world's most complex simulations and AI models—solutions that save costs and lives. That became my unique edge: using scientific computing paradigms to develop real-world applications that address real-world challenges.
[00:03:49]
Ken: As I said, it's quite an interesting origin story, so we like to ask that question. Let's jump right in and kick things off. Could you briefly introduce Phantasma Labs and the vision behind founding the company? What gap in the market were you looking to address?
[00:04:05]
Rama: Great. We envisioned building Phantasma Labs with the insight that there are several real-world use cases where AI can be leveraged without big data. This comes from my previous roles at companies where I used simulations instead of real-world data to train AI models. When I looked at the market, there were many opportunities to create robust AI solutions for traditional enterprises that don't have big data.
We founded Phantasma Labs to bring this proven technology to companies that essentially don't have data. To achieve this, we started leveraging reinforcement learning methodologies. The key here is that when we founded the company, this idea was a contrarian. In 2017-2018, AI hype was at its peak, especially around supervised learning approaches, and everyone thought that you needed vast amounts of data to build AI models. We took a different approach, saying, "You don't need big data to train these models."
Initially, we pitched our idea to automotive clients building autonomous cars and demonstrated that this approach works well—training models through simulations rather than relying on big data. This helped many enterprises catch up with companies that had large datasets. With that success, we transitioned to the industrial market.
We realized this is where our technology can have the most significant impact. It's a large market, and many factories and companies are looking to benefit from AI but don't have the necessary data. Our vision for Phantasma Labs is to combine simulations with AI technologies to provide AI solutions to classic companies lacking big data and the competence to build these models.
[00:06:01]
Ken: Phantasma Labs is revolutionizing production planning with AI-driven solutions. Could you briefly explain how reinforcement learning works with your platform and what makes it more efficient than traditional planning systems?
[00:06:15]
Rama: That's an excellent question our customers ask us frequently. Our reinforcement learning methodology differs from classical planning solutions in several key ways. First of all, it's the speed of execution. Our planning solution at the customer's end is typically 100 to 200 times faster than classical solutions. This is because we take the computational burden off the customer. We handle the training side, meaning our models are exposed to various planning scenarios before they're deployed to the customer.
Secondly, unlike traditional planning solutions that rely on operations research—a more heuristic approach—our models don't need to recompute everything from scratch for a new planning scenario. Take a factory, for instance: you're executing a plan, and something unexpected happens—machines break down, or staff aren't available. Typically, with classical approaches, you need to generate an entirely new plan because that's how the mathematical models work.
However, with reinforcement learning, we don't need to do that. Our model can efficiently move to the following optimal solution without disrupting the rest of the plan. This means our planning is faster and more adaptable. Reinforcement learning is also known for providing globally optimal solutions, whereas classical planning solutions often don't achieve that optimization level. As a result, we can generate highly effective planning outcomes that optimize manufacturing systems.
[00:08:08]
Ken: As I describe your company, I've referred to you as AI planning and scheduling versus advanced planning and scheduling. Still, of course, the same APS TLA, and so it resonates with people pretty well, and indeed, the results speak for themselves. I know that many AI-driven platforms rely heavily on big data. Now, you guys have taken a different approach. Can you explain how your technology bypasses the need for the traditional massive data sets and why this is a game changer, especially for small to medium enterprises or mid-sized manufacturers?
[00:08:45]
Rama: Right. This has to do with the underlying technology, which is reinforcement learning. The fact that we don't need big data comes from the environmental side of the reinforcement learning approach.
If you think about reinforcement learning, you have the "environment" where we stage different scenarios, and the "agent" learns from the impact of these scenarios, figuring out the best possible course of action. What we do is generate millions of scenarios at scale. If you were to gather data from millions of real-world scenarios in factories, it could take anywhere from six months to several years, depending on the complexity and size of the factory. But because we leverage modern computing paradigms, we've developed a unique scenario generation toolkit. This toolkit simulates various scenarios, including unforeseen situations a factory may not have encountered.
This means that factories don't need to wait for big data or collect it from real-world operations to utilize AI technologies. Another critical factor is our ability to accurately model processes within the environment. You can't apply reinforcement learning effectively without precise simulations, which requires much expertise and domain knowledge.
I also want to emphasize that this is not the same as training models with synthetic datasets. In our approach, the model learns directly within the simulation, a real game-changer for small and medium enterprises (SMEs) and mid-sized manufacturers.
For these companies, obtaining the necessary data to train models without simulation could mean investing millions upfront and waiting two to three years to gather enough data. We shrink that timeline to three to six months and reduce data collection costs by up to 80%. Additionally, we leverage technologies like knowledge distillation, which allows us to transfer learning from one set of scenarios to new ones, making the model exponentially smarter with each new scenario. This "curriculum learning" approach enables the agent to learn continuously and adapt to new situations without starting from scratch each time.
[00:11:47]
Ken: I keep returning to the idea of an origin story, and it's particularly interesting for you guys. Before founding Phantasma, as we mentioned, you had an impressive career working on large-scale simulations at SL Rasch and MAN Diesel & Turbo. How did those experiences shape the development of Phantasma today?
[00:12:07]
Rama: I think there are many similarities between simulations and AI, though they work in different directions. In simulations, you provide a set of inputs and get an output. But with the AI models we build, it works the other way around—you have a desired output and want to determine the most optimal inputs that lead to that output.
My work in simulations helped me understand where simulations are strong and where AI can complement their limitations. For example, at MAN Diesel & Turbo in 2012, we were already building what we now call AI models, though back then, they were referred to as meta models. Even before the AI boom, we saw the impact of such models on real-world applications.
This experience helped us in two key areas: first, it gave us the knowledge of the tools, infrastructure, and technology needed to build enterprise-grade software that integrates both AI and simulations. Second, it instilled a strong belief in the impact of these technologies. I've seen AI and simulations save lives.
For instance, before we did the Mecca pedestrian simulations at SL Rasch, there were frequent stampedes, and people were losing their lives. Through simulations and AI, we were able to prevent stampedes, even with 2 million people moving at the same time. No more stampedes have occurred since then, which is the kind of impact we aim to replicate with Phantasma Labs.
[00:13:50]
Ken: You've gained much initial traction in Germany, and now, with our recent investment and co-investors, you're looking to expand into the US. What challenges do you foresee in scaling to this new market?
[00:14:10]
Rama: Absolutely. We're excited to have Momenta and other investors on board, as this will help catalyze our expansion into new markets. We see four key challenges in expanding to the US market.
First, the US is a very traditional market, so we'll likely need a local sales force to address its specific needs. It's a huge market, and understanding those needs will be crucial.
Second, the market is fragmented in terms of digital maturity. Even within the SME and mid-cap segments, our unique selling proposition (USP) has to be broad enough to address a wide range of needs, which can vary significantly across different sectors.
Third, the US market is highly localized and geographically specialized. For example, Detroit is heavily focused on automotive, while Seattle is strong in aerospace. Their needs, tools, and indicators are different. We must integrate with each region's ecosystem and partners to provide the best solution.
Finally, we need to find a vertical market where we can establish a foothold and, from there, expand to other verticals and regions. Overcoming these challenges will be critical for our success in the US.
[00:15:54]
Ken: Since Rockwell Automation backs us as our anchor LP, there's always an opportunity to partner in these areas. When you look at large MES (Manufacturing Execution System) providers, there's a potential growth path with OEMs, especially in reaching SMEs with so much opportunity.
We all know the manufacturing sector has slowly embraced digital transformation, particularly AI. What are the most significant barriers holding the industry back, and how do you think Phantasma Labs will overcome them?
[00:16:47]
Rama: Right. We've spoken to several customers across different markets and geographies over the past few months and the last year. Despite the variety, we've noticed some consistent obstacles when approaching customers in this market.
First, there are misconceptions about AI. Many people in the industry believe AI will take their jobs or their data will be misused. Additionally, management tends to shift focus on what kind of AI technology they want to implement. Before ChatGPT and large language models (LLMs), everyone was focused on using AI for productivity, maintenance, forecasting orders, and pricing. However, once the ChatGPT movement gained traction, management asked, "Can you build ChatGPT for us internally?" We often had to explain that it wasn't feasible for their needs. There's a significant gap between expectations and what AI can realistically deliver.
Second, many customers worry about the upfront costs of AI solutions and the uncertainty of return on investment (ROI). This concern stems from unmet promises made by AI technologies in the past. People expected quicker results, but some solutions take time to build and show returns. We've seen that many past AI projects, which were merely proof-of-concept (POC), are now evolving.
Overall, misconceptions about AI have been a crucial barrier. Another obstacle is the political inertia within industries, primarily driven by legacy tools. Many factories already have tools and are reluctant to replace or expand their capabilities. This reluctance also stems from resistance to change within factories. People prefer to stick with the familiar rather than train staff on new tools. Additionally, there's a desire to keep humans at the center of operations. The misconception that AI will replace jobs creates hesitation in pushing these solutions forward.
Lastly, we see a disconnect between AI solution's perceived and real value. Some customers overestimate the benefits, while others don't fully appreciate the value they're receiving. To overcome these barriers, we help our customers understand the "before and after" of using AI solutions like ours. We demonstrate how our solutions improve key performance indicators (KPIs), reduce costs, and save time. We also aim to reduce upfront costs and deliver ROI within six months. While we've made progress, there's still work to address these challenges at Phantasma Labs and in the broader market.
[00:20:10]
Ken: One thing we appreciate about your approach is your focus on small to medium enterprises (SMEs), which aligns with other companies in our portfolio. How are you targeting these companies, and how has that approach worked in practice?
[00:20:27]
Rama: As you mentioned, we target SMEs and mid-cap companies, which comprise about 80% of our market. We offer a unique value proposition to make it easy and impactful for them to adopt our technology.
First, we reduce the upfront cost and onboarding time so our customers don't have to deal with long development cycles. We can deliver our product within four weeks, which allows customers to quickly see the impact and determine the value of using it long-term. This speed of adoption is unique to our technology and execution, and it's been very well-received.
Second, our approach is tailored to the current needs of this underserved market. Many of our customers want to use AI but don't have the data or resources to do so. We tell them, "You don't need to change how you operate. We can elevate your current setup to the next level with AI-based planning solutions." This resonates with customers because they often need extensive preparation for our solution. However, our technology doesn't require big data and integrates easily with existing tools. This makes it easy for them to adopt and use our products in the long term.
[00:22:18]
Ken: Given your extensive background in computational mechanics and AI, I'm curious—what technology advancements do you foresee having the most impact on manufacturing in the next five to ten years?
[00:22:29]
Rama: I'm particularly excited about innovation in computing, and I believe one of the most significant shifts will be in the speed and cost of training and deploying models. I sometimes controversially predict that at some point, our most loved computing paradigms will be universally disrupted, leading to much faster computing technologies. This will allow us to train models much quicker, which is essential because it directly affects how fast and cost-efficiently we can deliver models to our customers.
Second, industries are moving away from focusing on big data alone and shifting toward algorithmic development. Traditionally, customers believed they needed vast amounts of data to drive innovation, but as our understanding of different domains grows, I think the emphasis will shift to developing smarter algorithms. This means codifying human knowledge and intelligence into AI systems rather than just feeding AI more data and relying on black-box models.
Third, AI technologies will increasingly become part of a human feedback loop. Models will work alongside humans instead of just learning offline, with feedback helping them improve. This integration will allow AI to give better, more explainable results. We'll see significant advancements in this area within the next five to ten years, especially with the acceleration of computing speeds.
[00:24:11]
Ken: As we wrap up, I'm curious—how do you stay ahead in such a rapidly evolving space? What books, podcasts, or thought leaders do you turn to for inspiration and to keep your knowledge current?
[00:24:26]
Rama: I'm a voracious reader and enjoy reading books by leaders in the field. I'm reading one by Kence Anderson, who wrote about designing autonomous systems for industry. It's a great resource for anyone leading the AI revolution. I also listen to several podcasts, including Industrial AI, which is sponsored by Siemens. It offers a futuristic perspective on how AI will shape the next innovation cycle in manufacturing.
In addition to reading and listening, I stay involved with working groups developing the next systems for industrial domains, including data spaces and model standardization. These groups keep me connected to the latest advancements and help me maintain my edge.
[00:25:37]
Ken: Excellent. Rama, thank you again for sharing your time and insights today. It's been a great conversation.
[00:25:44]
Rama: Thank you so much, Ken. I appreciate Momenta's support and am excited to be part of this journey as we drive the AI revolution in the industrial space.
[00:25:58]
Ken: Absolutely. We're very proud to have Phantasma Labs in our industrial impact portfolio. This has been Ramakrishna Nanjundaiah, founder and CEO of Phantasma Labs.
Thank you for listening. Please join us for the next episode of our Industrial Impact podcast. We wish you an impactful day.
You've been listening to the Momenta Digital Thread podcast series. We hope you've enjoyed the discussion, and as always, we welcome your comments and suggestions. Please visit our website at momenta.one for archived versions of podcasts and resources to help you on your digital industry journey. Thank you for listening.
[The End]
Connect with Rama Nanjundaiah on LinkedIn!
Maintaining a competitive edge
About Phantasma Labs:
Phantasma Labs builds AI-based decision-making software to optimize operational planning and scheduling processes without the need for historical company data. Our AI models understand the impact of various parameters and their effects on the KPIs. They can thereby suggest KPI-driven optimal decisions for planners and operators for various scenarios occurring in manufacturing firms and enable dynamic (re)planning in a highly efficient way. This makes factories understand the impact of various what-if scenarios and black swan events, allows them to respond to changes in their planning parameters quickly, and significantly increases their process efficiency – no big data is needed.